Complete Guide to Image Sensor Specs for Optimal Performance
- Regami Solutions
- Jan 17
- 4 min read
Updated: Mar 11
When selecting the right image sensor for your application, understanding the key terminology is essential. By using these terms, you can better understand the image quality that sensors produce and make sure that the right sensor is selected for your needs.
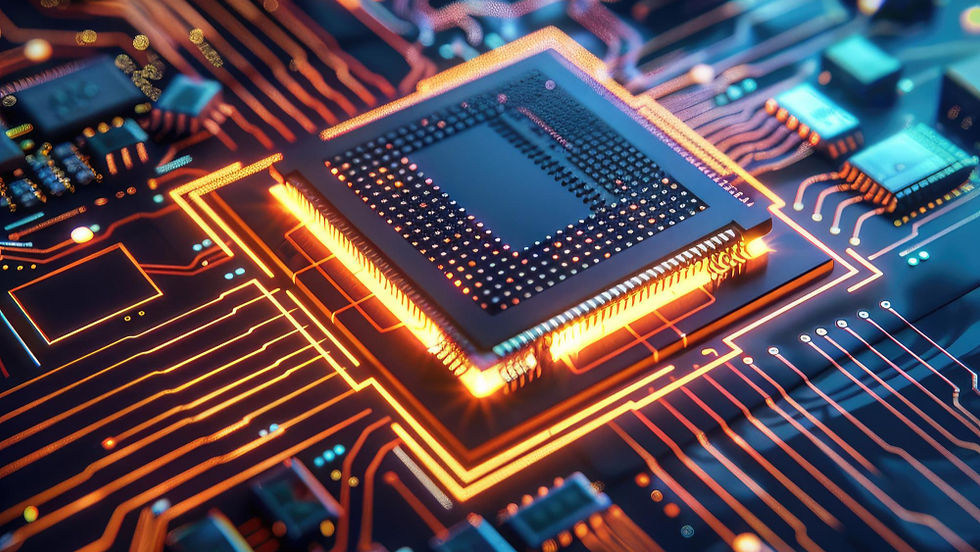
Below, we explore the most important terms related to image sensors, including sensor format, pixel size, resolution, signal-to-noise ratio (SNR), responsiveness, and dynamic range.
Need expert advice on selecting the right camera for your application? Reach out to our Camera Engineering Services today to discover the ideal camera solution for your project!
Significant Features of an Image Sensor
Several specifications and terms help evaluate the quality of an image sensor. These include sensor format, pixel size, resolution, signal-to-noise ratio (SNR), responsiveness, and dynamic range. Understanding each of these terms and how they impact image quality can guide you toward the most suitable sensor for your application.
Sensor Format and Its Impact on Image Sensor Performance
One of the most common specifications found on datasheets for image sensors is the sensor format, which could be listed as 1/3”, 1/4”, or 1/2.3” and so on. This format indicates the diagonal measurement of the active image area on the sensor.
The term "inch" refers to the optical form factor, originally based on tube cameras using a 1" deflection coil for image capture. This standard 1" optical format is equivalent to 16mm.
The sensor format plays an essential role in determining how much light a sensor can capture and the overall field of view, influencing image quality. Larger sensors, like 1/2.3” or 1”, tend to offer better low-light performance and image clarity.
Pixel Size: More Light, Better Image Quality
Pixel size is another critical factor when evaluating image sensor quality. A pixel is the smallest element on a sensor that captures light and converts it into an electrical signal, eventually creating the image. Larger pixels are more capable of gathering light, resulting in better images, especially in low-light conditions.
However, technological advancements have led to improvements in sensor design, allowing for higher-quality images even with smaller pixels.
This is where sensor architectures like front-side illumination (FSI), back-side illumination (BSI), and BSI II come into play. These designs optimize the way light is captured and help maintain image quality even with smaller pixels.
Resolution: Do More Pixels Mean Better Quality?
Resolution refers to the total number of pixels on an image sensor. More pixels typically mean that the sensor can capture finer details, which is important in applications like optical character recognition (OCR) or wide-angle image capture. Higher-resolution sensors are often preferred for applications that require sharp, detailed images.
However, the relationship between resolution and image quality is not always straightforward. A higher resolution can result in smaller pixels, reducing light sensitivity.
In low-light environments, a lower-resolution sensor with larger pixels may offer better image quality. However, advancements in pixel technology now allow modern sensors to achieve higher resolutions without compromising low-light performance.
Signal-to-Noise Ratio (SNR): A Critical Measure of Image Clarity
The signal-to-noise ratio (SNR) is one of the most important metrics for evaluating image sensor quality. SNR calculates the proportion of undesired noise (random fluctuations in the sensor's signal) to the desired signal (light picked up by the sensor). A higher SNR means better image quality, as the sensor captures more of the signal and less of the noise.
In low-light environments, the SNR at lower photon levels per pixel is crucial, while in brighter environments, the SNR at higher photon levels is considered. The SNR curve helps assess sensor performance under specific lighting conditions, ensuring you select the right sensor for your needs.
For different use cases, the SNR curve provides valuable insight into how the sensor performs in various lighting conditions.
Responsivity: How Sensitive Is Your Image Sensor to Light?
Responsivity refers to how efficiently an image sensor converts incoming light into an electrical signal. The higher the responsivity, the less light is required to produce a clear image. This makes responsivity an important specification when evaluating sensors for low-light applications.
Responsivity is depicted as a curve showing the electrical output at various light wavelengths.
It helps assess sensor performance under different lighting conditions, making it crucial for applications like night vision or infrared imaging. A sensor with high responsivity ensures high-quality images, even in low-light or challenging environments.
Dynamic Range: Capturing Both Bright and Dark Details
Dynamic range is a crucial factor when choosing an image sensor. It refers to the sensor's ability to capture details in both the brightest and darkest areas of an image.
A higher dynamic range means the sensor can retain more detail in highlights and shadows, leading to more balanced and realistic images.
For example, an HDR image preserves more detail in both bright and dark areas compared to a lower dynamic range image. This is essential for applications like outdoor photography or surveillance, where scenes with varying light levels need to be captured accurately.
Our Vision Engineering team specializes in delivering customized solutions for healthcare, robotics, automotive, and more. Get In Touch and leverage our Expertise for your Business!
Choosing the Right Image Sensor for Your Needs
When selecting an image sensor, it's important to consider key specifications like pixel size, sensor format, resolution, SNR, dynamic range, and responsivity to ensure optimal image quality. This is critical across various industries, such as healthcare, retail, automotive, and aerospace, where the right sensor can significantly impact the precision and quality of results in applications like medical imaging, autonomous vehicles, and facial recognition.